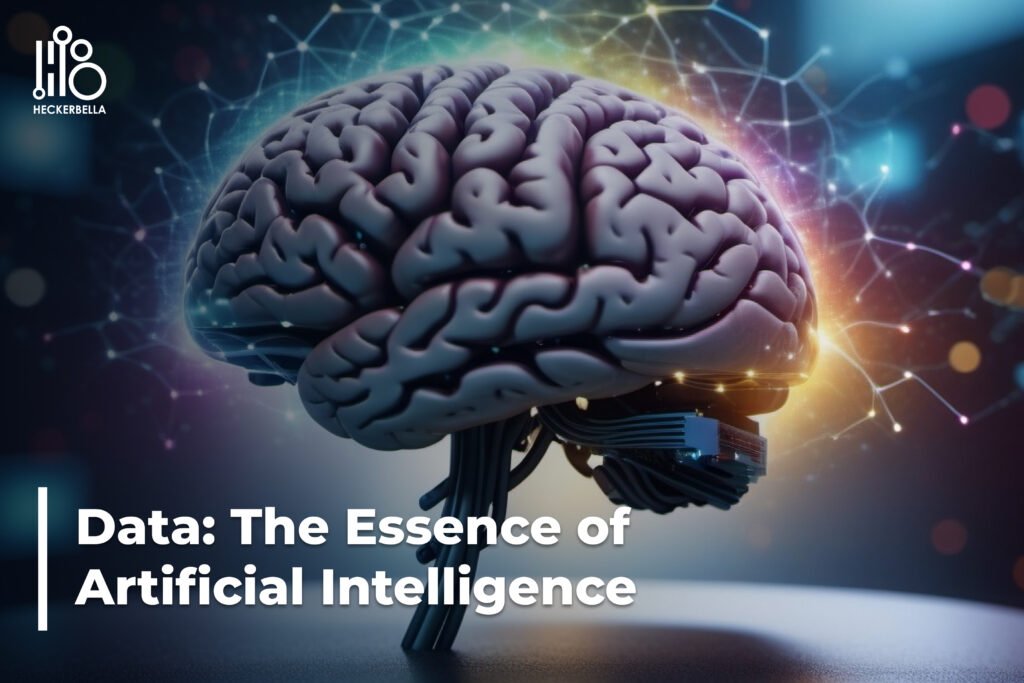
Artificial intelligence (AI) has captured the world’s imagination. From self-driving cars to virtual assistants, AI promises to revolutionize the way we live and work. But beneath the surface of these marvels lies a crucial element: data. Data isn’t just the fuel that propels AI; it’s the very foundation upon which it’s built.
Learning from Experience: The Power of Data
Think of a child learning a new language. The more words they hear and practice using, the better they understand the language’s nuances. Similarly, AI systems learn and improve through exposure to data. This data provides the raw material for AI to identify patterns, make connections, and ultimately perform tasks.
The Richer, the Better: Quantity and Quality of Data
The amount and quality of data play a critical role. Large, diverse datasets empower AI to identify intricate patterns and relationships within vast information landscapes. This translates to highly accurate predictions, personalized experiences, and the ability to automate complex tasks with exceptional efficiency.
Beyond Spreadsheets: A Spectrum of Data for AI
AI doesn’t solely rely on the traditional, neatly organized data found in spreadsheets. The real world is messy, and AI is increasingly leveraging various types of data for a more holistic understanding:
- Structured Data: Customer information, financial records, and sensor data from connected devices provide a solid foundation for AI analysis.
- Unstructured Data: Images, videos, social media posts, and even text in local languages offer a treasure trove of insights. Techniques like Natural Language Processing (NLP) allow AI to extract meaning from this unstructured data, enriching its understanding of the world.
Quality Matters: The Importance of Clean Data
Quantity is important, but it’s not everything. The more data an AI system is trained on, the better it performs, but only if the data is clean and accurate. High-quality, accurate, and unbiased data is essential for effective AI training. Dirty or flawed data can lead to biased or inaccurate AI models that produce unreliable results.
Real-World Examples: The Power of Data in Action
Consider these real-world applications where data fuels AI’s capabilities:
- Smart Farming: AI analyzes weather patterns, soil conditions, and historical yields to optimize crop planting and resource allocation, maximizing agricultural output.
- Fraud Detection: AI algorithms analyze financial transactions in real-time, identifying patterns indicative of fraudulent activity, protecting businesses and consumers alike.
- Personalized Learning: AI tailors educational experiences to individual student needs by analyzing learning styles, strengths, and areas for improvement.
Challenges and Considerations: Responsible AI Development
While the power of data in AI is undeniable, challenges arise that require careful consideration:
- Data Bias: AI models can perpetuate biases present in the data they’re trained on. This can lead to discriminatory outcomes if not addressed through diverse and representative training data.
- Data Privacy: As AI interacts with more data, concerns about data privacy and security become paramount. Robust data security measures and clear regulations are essential to protect sensitive information.
- Explainability of AI: In some cases, it can be difficult to understand how AI arrives at its decisions. This lack of explainability can raise concerns about transparency and accountability, particularly in high-stakes applications.
Building a Better Future: The Road Ahead
Imagine AI as a powerful tool, and data as the raw materials needed to craft it. The quality and responsible use of these materials will determine the outcome. By using data thoughtfully, we can unlock AI’s potential to create a future that’s not just smarter, but fairer and more beneficial for everyone. This means working together on several fronts:
- Data Governance: This involves creating clear guidelines for how data is collected, stored, and used. We need to make sure it’s done ethically and with people’s privacy in mind.
- Data Literacy: Everyone needs to understand the role of data in AI. This empowers individuals and organizations to participate responsibly in the AI revolution.
- Collaboration: Bringing together AI developers, data scientists, and ethicists from the very beginning is key. This ensures fairness, transparency, and accountability are built right into AI from the ground up.
The potential of AI is exciting, but it relies on a solid foundation: data. By using data responsibly, we can ensure that AI becomes a powerful tool that helps us tackle challenges and improve lives across industries.
References
https://www.ibm.com/blog/structured-vs-unstructured-data/
https://www.ibm.com/blog/structured-vs-unstructured-data/
https://www.sciencedirect.com/topics/agricultural-and-biological-sciences/precision-agriculture
https://www.sciencedirect.com/science/article/pii/S2405896322017153
https://www.emerald.com/insight/content/doi/10.1108/ITSE-01-2023-0007/full/html
https://www.europarl.europa.eu/RegData/etudes/BRIE/2019/640163/EPRS_BRI%282019%29640163_EN.pdf